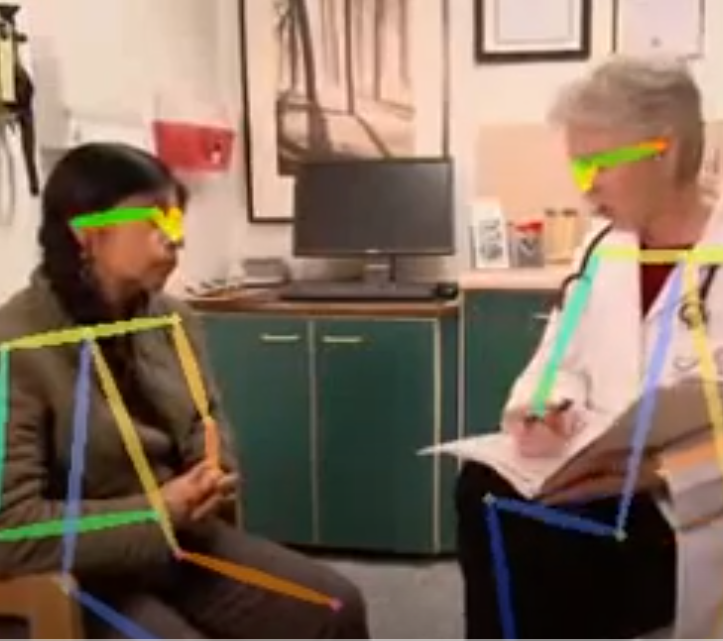
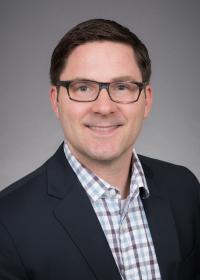
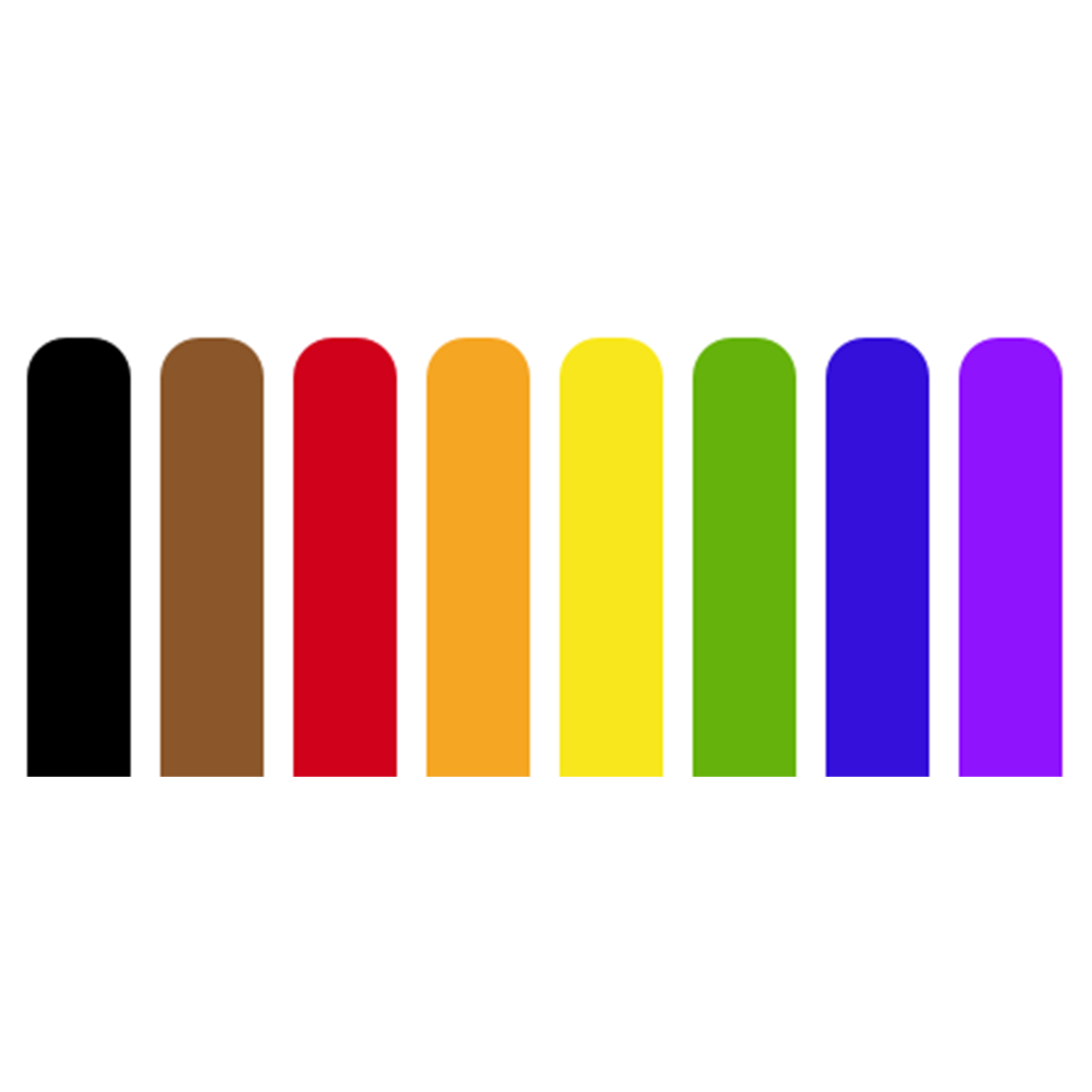
UnBIASED patient-provider interactions

Implicit bias in medicine
Our physicians prioritize creating an inclusive and respectful clinical environment to better support and care for their patients – this includes actively looking to identify and mitigate implicit biases in clinical settings.
As described by the UW IM Resident Diversity Committee, “bias is an inherent part of human behavior that must be intentionally and proactively addressed in order to rectify injustice in medicine, and to deliver equitable, high-quality care.”
What does implicit bias look like?
Bias in healthcare can manifest in different ways that affect patients. This includes during individual interactions (e.g., dismissiveness, making assumptions about behavior, blaming patients, stereotyping, less patient-centeredness, lower rates of referral) and structural characteristics (ex. lack of diversity in clinic staff, lack of representation in promotional/education materials).
Research has consistently shown that the presence of implicit bias (immediate, unconscious associations) in clinical encounters negatively impacts patient-provider communication, trust in the medical system, care quality, and ultimately contributes to health disparities and inequities.
Disparities in treatment decisions for issues like pain, cardiovascular conditions, and cancer have been associated with implicit bias. The impact of implicit bias has been found to weigh more heavily in clinical decisions during situations that have higher ambiguity or subjectivity, incomplete information, or compromised cognitive load (due to fatigue, for example).
“As providers, we are human, which means our brains make unconscious associations. We need to be aware of this potential and need to talk openly about how implicit associations can impact patients and patient care,” says Dr. Brian Wood, associate professor (Allergy and Infectious Diseases).
Implicit bias between providers and patients
Wood is a co-investigator on the UnBIASED (Understanding Biased patient-provider Interaction and Supporting Enhanced Discourse) Project, led by Dr. Andrea Hartzler, associate professor (UW Department of Biomedical Informatics and Medical Education) and Dr. Nadir Weibel, associate professor (Computer Science and Engineering at UC San Diego), and funded by the National Library of Medicine at NIH (R01 LM013301).
The goal of the project is to develop an automated tool that can be integrated into the clinic setting or training environment to help raise clinicians’ awareness of moments of implicit bias in their interactions with patients.
The project combines input from members of traditionally marginalized groups, including BIPOC and LGBTQ+ individuals, to learn about biased behaviors that manifest in healthcare settings and to better understand the impact on patients.
The UnBIASED team has also partnered with clinicians at Harborview Medical Center and UC San Diego clinics, who volunteered to have cameras installed in their exam rooms to record actual patient visits (with patient consent) and to have their interactions analyzed for potential indicators of bias.
Methods
Using audio and visual recordings of visits, and building on prior work that established an association between non-verbal communication cues (e.g., interruptions) and specific social signals associated with implicit bias, such as verbal dominance, the UnBIASED team is using an artificial intelligence (AI) approach that assesses signals that have been associated with positive or negative moments in medical encounters.
The goal, according to Hartzler, is “to use informatics approaches like machine learning to help automate the assessment of patient-provider interaction, which has in the past has relied on manual review by human observers. We are teaching computers to do this assessment, which requires collecting lots of examples of patient-provider interactions."
To accomplish this, the team is using computational sensing to identify a number of verbal and non-verbal cues (e.g., interruptions, talk time, turn taking, tone of voice, eye contact, nodding, posture, and others) and collecting feedback from providers and patients on their interpretation of pivotal moments during the encounters.
Sample of the visual the AI software uses (actor portrayal); there is no accompanying audio
They are simultaneously co-designing (with providers) methods to deliver feedback to clinicians on the communication patterns that are detected by the AI system. Potential feedback mechanisms include a dashboard that illustrates differences in communication patterns over time or between patients of different demographics, guided reflection, or “digital nudges” during encounters. The goal is to increase clinician awareness of communication patterns, both positive patient-centered interactions and opportunities for improvement.
Says Wood, “We are exploring options to develop continuing education workshops that combine the UnBIASED automated assessment feedback tool with standardized patient visits, and potentially integrating the feedback tool into medical school and training programs, with the goal of motivating personal reflection and aspirational change.”
tackling implicit bias
As stated by Dr. Janice Sabin, research associate professor (UW Department of Biomedical Informatics and Medical Education) in a recent article in Science, “We all have hidden biases. It is how our minds work. We make and harbor automatic associations.
Research suggests that these hidden implicit biases can affect healthcare communication, treatment decisions, and patient trust, and contribute to well documented health disparities among people of color, women, members of the LGBTQ+ community and other historically marginalized groups.”
Sabin is a co-investigator on the UnBIASED project, and has created the “Equity, Access, and Inclusion in Hiring” training module at the University of Washington to reduce implicit bias in hiring (required for all members of search committees within the Department of Medicine).
Sabin also recently published a landmark perspective piece on tackling implicit bias in healthcare in the New England Journal of Medicine.
Overall, the goal of UnBIASED is to detect and raise awareness of hidden bias in patient-provider communication, give providers insights into positive and negative moments and how they interact with patients from differing backgrounds, and improve the patient experience in healthcare. Read more about UnBIASED and similar ongoing research from Science.