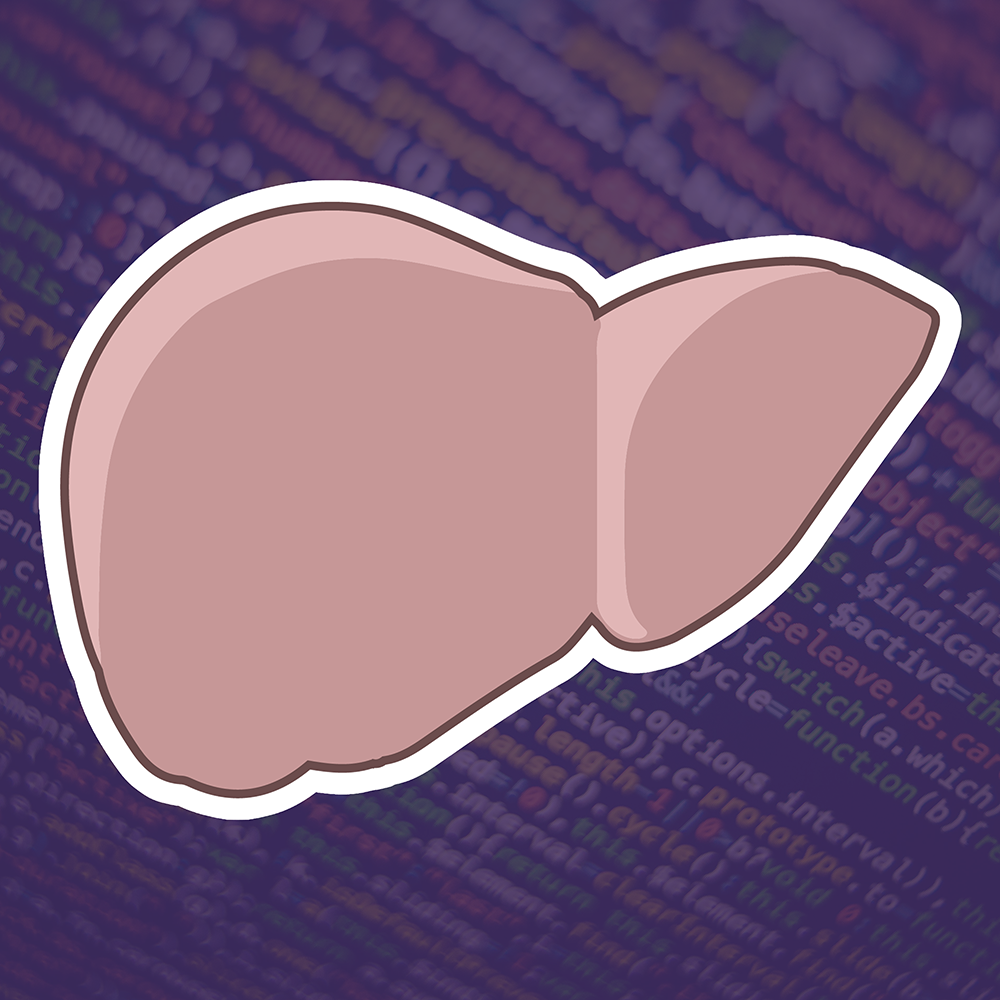
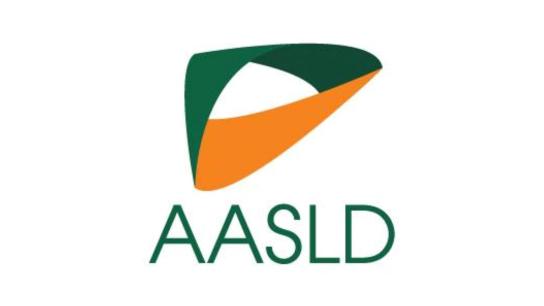
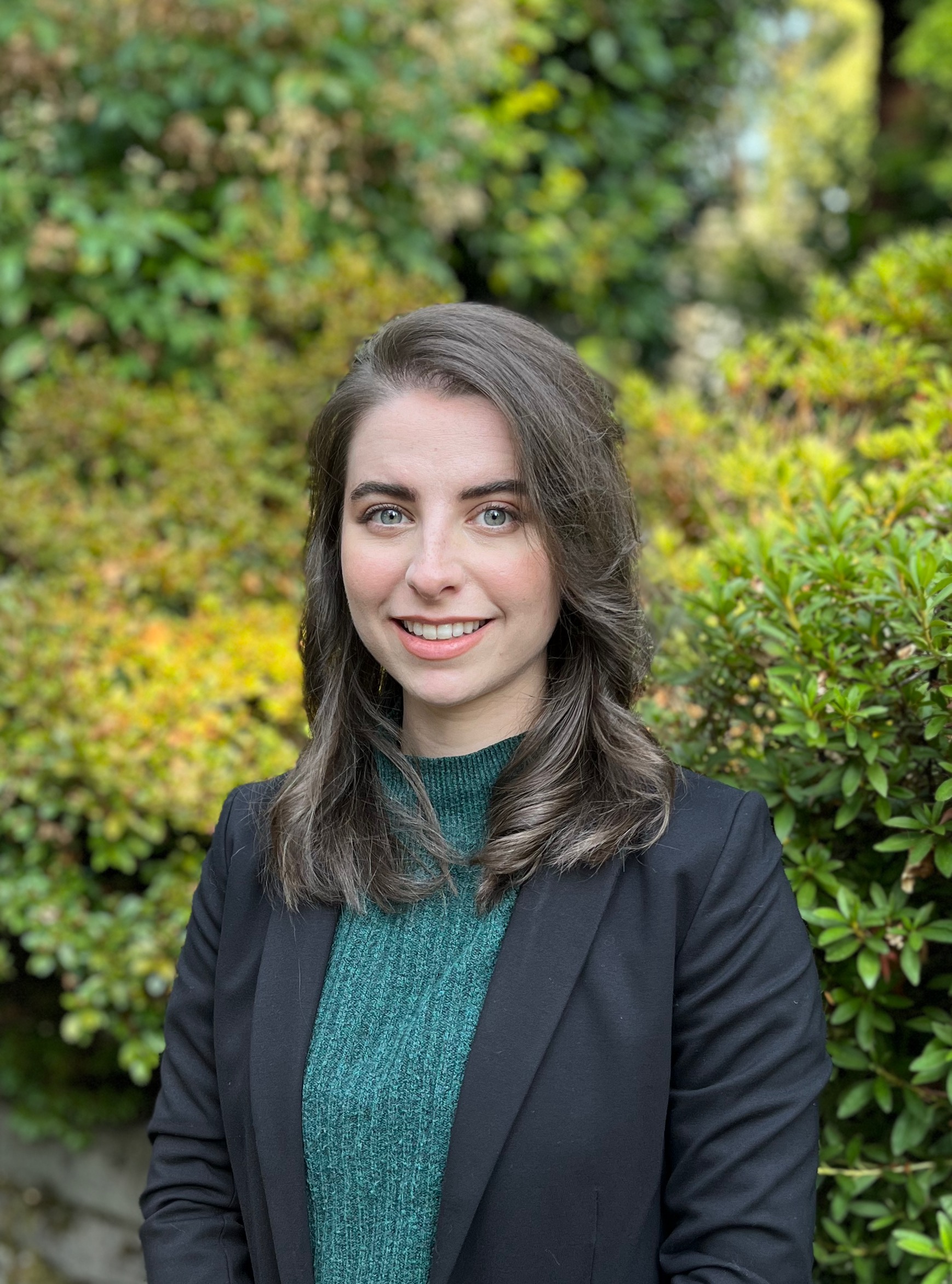
AI may help uncover hidden liver disease

Machine learning helps identify MASLD
New research from the Carr Lab demonstrates an iterative natural language processing AI algorithm applied to data from radiology reports successfully identified patients with metabolic dysfunction–associated steatotic liver disease (MASLD).
What is MASLD
MASLD, one of the most common forms of chronic liver disease, occurs when fat builds up in the liver causing inflammation and potentially leading to cirrhosis or severe fibrosis. Early diagnosis and treatment are among the most important factors for preventing disease progression and serious complications.
Physicians traditionally diagnose MASLD based on risk factors obtained from patient history, imaging and blood test findings. However, many patients with MASLD are asymptomatic and undiagnosed, sometimes only receiving a diagnosis once their condition has progressed.
Diagnosis aided by AI
The Carr Lab
The Carr Lab, led by Dr. Rotonya Carr, associate professor and head (Gastroenterology), investigates the causes of and treatments for fatty liver diseases, including MASLD (nonalcoholic fatty liver disease) and alcoholic-associated liver disease (ALD).
A Carr Lab researcher team, led by Dr. Ariana Stuart, UW internal medicine resident, developed a study to test an iterative natural language processing AI algorithm in identifying MASLD diagnosis in a broad demographic of patients.
“I'm very excited to be part of Dr. Carr's team working to utilize machine learning to address gaps in our healthcare system,” Stuart said. “Machine learning is a promising tool to help augment clinical practice to avoid missing potentially critical findings, or even to identify clinical patterns or connections that were previously unrecognized.”
These types of AI systems have the ability to analyze narrative language and additional data inputs to generate correlations, and can be refined over repeated cycles of analysis and feedback.
The algorithm developed for this study used MASLD criteria from the American Association for the Study of Liver Disease to analyze radiology reports from multiple Seattle area medical centers. The algorithm predicted MASLD diagnosis for cases in which the criteria were met and the radiology report indicated likely MASLD. Two physicians then manually reviewed the medical records to validate the results.
The study found that the algorithm had an accuracy of 88% in identifying patients with imaging findings consistent with MASLD. Notably, only 17% of this group had an MASLD ICD diagnosis and 26% had been seen by a gastroenterologist or hepatologist. These findings were recently presented at The Liver Meeting held by the AASLD.
“Our primary finding - that only ~20% of patients meeting criteria for MASLD had been diagnosed - reflects how challenging it can be to keep track of all the data collected on patients in the EHR. People should not interpret our findings as a lack of primary care training or management,” Stuart said.
“Instead, our study shows how AI can complement physician workflow to address the limitations of traditional clinical practice.”
The team plans to expand the study to a larger cohort and implement a quality improvement initiative for primary care physicians on recognizing risk and promoting interventions for early indicators of MASLD.
A benefit to patients
This study helps to demonstrate how AI methods can be used to improve clinical assessment and inform decision making to benefit patients. This potential is especially acute for complex conditions such as MASLD, which often occurs without clear symptoms, as it may offer a significant opportunity for earlier detection and intervention.