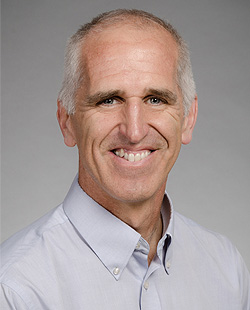
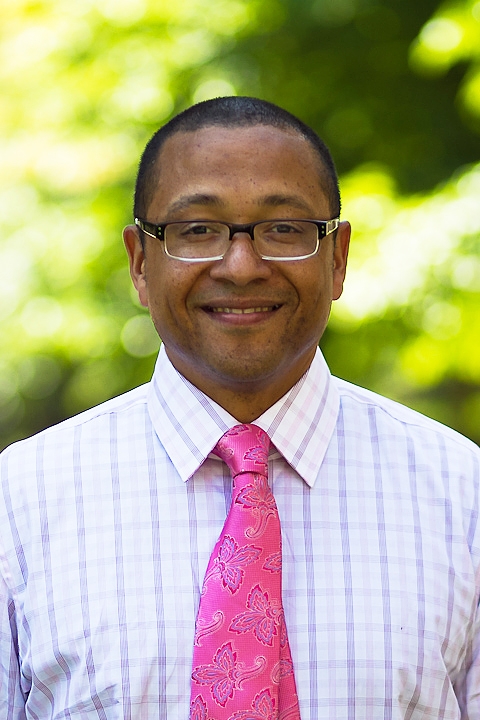
Using AI for Tuberculosis Classification Using Wearable Data

The UW Population Health Initiative announced the award of five, $100,000 artificial intelligence-focused pilot grants to support interdisciplinary teams of University of Washington researchers to develop preliminary data or proof-of-concept needed to pursue follow-on funding to scale their respective efforts.
Researchers from the Department of Medicine – Drs. Thomas Hawn, professor (Allergy and Infectious Diseases) and David Horne, associate professor (Pulmonary, Critical Care and Sleep Medicine) – and Dr. Shwetak Patel from the Allen School of Computer Science & Engineering received a grant for a pilot study that aims to use wearable sensors to classify TB infection based on cough characteristics in real-world settings.
According to the WHO, tuberculosis (TB) is the leading infectious disease-related cause of death, killing 1.5 million individuals each year and causing disease in 10 million. With the increasing ubiquity of connected technologies in developing countries there is an opportunity to use these tools to help diagnose and limit TB in a population.
Researchers recently created a model that distinguishes TB from non-TB coughs from smartphone recordings. Although these results are promising, this study and others are done in controlled lab settings and conditions. While this is useful in comparing different model performance to this type of data, it does not address the many nuances of real world data that would be needed to deploy outside controlled situations.
Wearables offer continuous unobtrusive monitoring and increased access to signals throughout the day, but come with additional signal noise.
The primary aim will be to collect continuous data with lab test ground truth to create an ML model diagnosing TB infection that is robust across situationally diverse conditions. Secondarily, researchers will explore the use of biometrics from Fitbit data and the use of generative AI as ways to create a more robust classifier.
With a robust pipeline, the proof of concept will be a stepping stone towards real time community deployable models that can allow for early diagnosis and notification to decrease TB transmission events and address TB control.